The Importance of Labeled Image Datasets in Modern Business
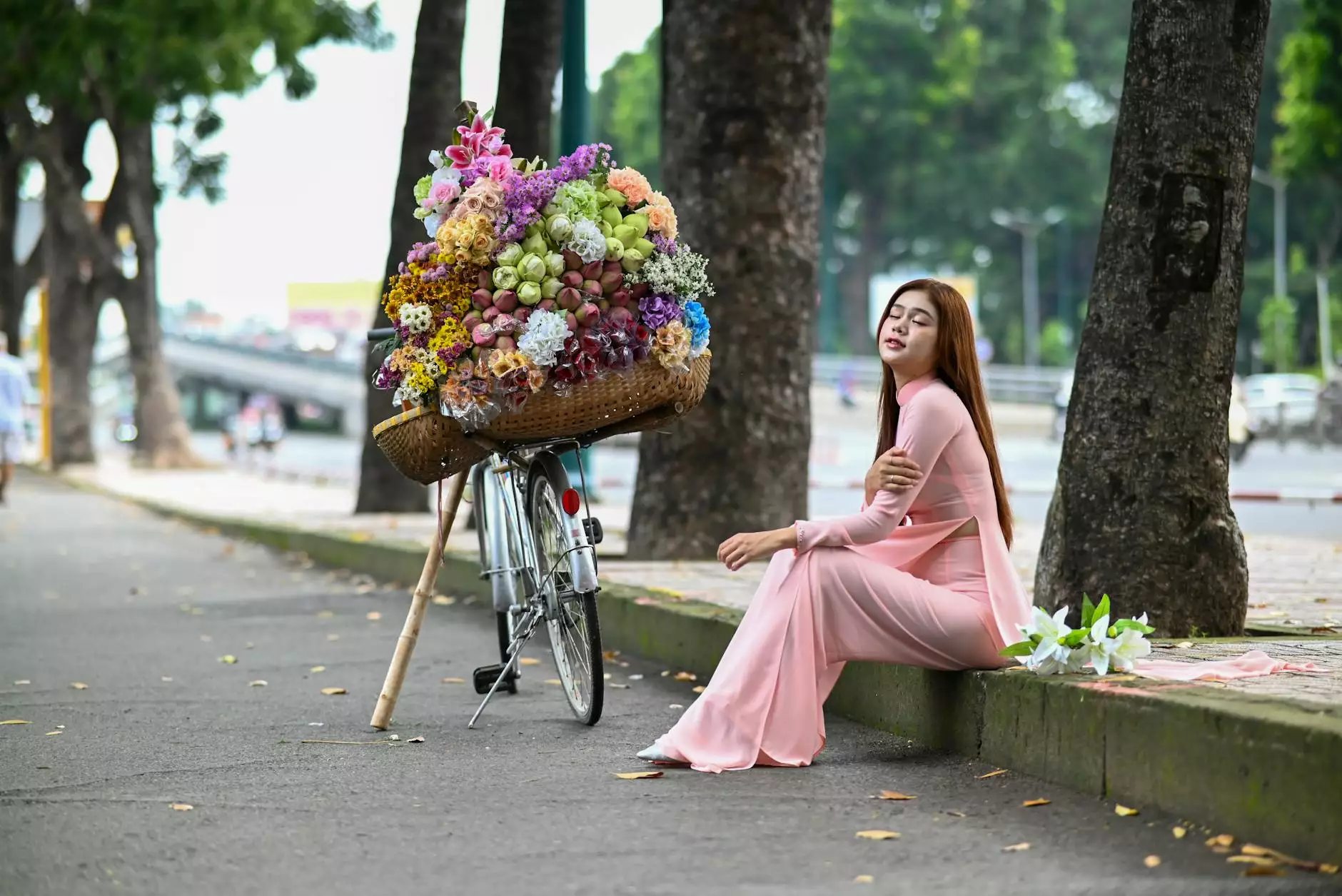
In today's digital age, businesses are increasingly relying on data-driven decisions to enhance their operations and gain a competitive edge. At the core of this data-driven strategy lies a valuable resource: labeled image datasets. These datasets not only form the backbone of artificial intelligence (AI) and machine learning (ML) models but also play a pivotal role in various industries ranging from healthcare to autonomous vehicles.
Understanding Labeled Image Datasets
Labeled image datasets are collections of images that have been annotated with relevant information. This can include identifying objects within the images, categorizing the images, or even providing context for certain features within an image. These labels are crucial for training machine learning models as they allow computers to learn from the data and make accurate predictions.
Why Are They Essential?
- Training AI Models: Machine learning models depend on high-quality labeled datasets to learn and generalize from examples. The accuracy of the model is directly tied to the quality and quantity of labeled data.
- Real-World Applications: Labeled image datasets are fundamental in training models for real-world applications such as facial recognition, medical imaging, and retail analytics.
- Enhanced Decision Making: Businesses can leverage insights from these datasets to make informed decisions, improving operational efficiency and customer satisfaction.
The Role of Data Annotation Tools
To create effective labeled image datasets, businesses require efficient data annotation tools. These tools streamline the process of labeling images, making it faster and more accurate. Keylabs.ai offers a sophisticated data annotation platform that serves this very need.
Key Features of Data Annotation Tools
- User-Friendly Interface: A well-designed interface allows users to annotate images with ease, enabling quicker turnaround times for projects.
- Flexibility: An ideal data annotation tool should support multiple forms of annotation, including bounding boxes, segmentation, and keypoint identification.
- Collaboration: Tools that support team collaboration can significantly improve productivity by allowing multiple users to work on the same dataset.
Applications of Labeled Image Datasets in Various Industries
The applications of labeled image datasets are vast and growing across various sectors. Here's a detailed look at how different industries are utilizing this technology:
1. Healthcare
In the healthcare sector, labeled image datasets are crucial for developing AI solutions that enhance diagnostic accuracy. For example, medical imaging tasks such as identifying tumors in x-ray or MRI images rely on annotated datasets to train models capable of recognizing abnormalities.
2. Automotive
The automotive industry is another area where labeled image datasets play a significant role. Self-driving cars utilize these datasets to understand their surroundings, recognizing pedestrians, traffic signs, and other vehicles, thus ensuring safe navigation on roads.
3. Retail
In retail, machine learning models trained on labeled datasets help in analyzing customer behavior through image recognition, affecting everything from inventory management to personalized marketing strategies.
4. Agriculture
Agricultural technology leverages labeled image datasets for precision farming. Drones equipped with imaging technology can assess crop health through image analysis, helping farmers optimize yields and resource usage.
Creating Effective Labeled Image Datasets
The process of creating effective labeled image datasets involves several critical steps:
1. Data Collection
Begin by collecting a diverse range of images that represent the scenarios or objects you want your model to understand. A comprehensive dataset will lead to better model generalization.
2. Data Annotation
Employ a reliable data annotation tool to label your images. Depending on the application, this could involve marking rectangles around objects, identifying points of interest, or even segmenting images into different regions.
3. Quality Assurance
Before using the labeled dataset, perform a quality assurance check to ensure that the annotations are accurate. Inaccurate labels can lead to poor model performance.
4. Iterative Improvement
After deploying your model, continue to collect feedback and improve your dataset iteratively. This feedback loop is essential for maintaining model accuracy over time.
Challenges in Labeled Image Dataset Creation
Despite the benefits, creating and maintaining labeled image datasets comes with its own set of challenges:
- Scalability: As the need for data increases, managing large datasets efficiently can become difficult.
- Cost: High-quality data annotation can be expensive, especially when outsourcing these tasks.
- User Expertise: Effective annotation requires skilled personnel who understand the context of what they are labeling.
Choosing the Right Data Annotation Platform
When it comes to selecting a data annotation platform, businesses should consider several factors:
1. Efficiency
Look for platforms that offer automation features to speed up the data annotation process without sacrificing quality.
2. Support for Various Annotation Types
Your platform should support a variety of annotation types to accommodate different needs depending on the nature of your images.
3. Integration Capabilities
A good data annotation tool should integrate seamlessly with your existing workflows and tools.
Why Keylabs.ai is a Leading Data Annotation Platform
Keylabs.ai stands out as a premier choice for businesses looking to create labeled image datasets. With its robust features and commitment to quality, Keylabs.ai helps streamline the data annotation process across industries.
Keylabs.ai Features
- Advanced Tools: Keylabs.ai provides advanced annotation tools that cater to various automation strategies, reducing project turnaround times.
- Collaborative Workflows: The platform supports team collaboration, allowing for concurrent work on different parts of the dataset.
- High Precision: With a focus on quality control, Keylabs.ai ensures that every annotated image meets the highest standards needed for effective model training.
The Future of Labeled Image Datasets
As the fields of AI and machine learning continue to evolve, the role of labeled image datasets will become even more significant. Innovations such as semi-supervised learning and self-supervised learning techniques may further enhance the efficiency of training models, meaning less reliance on human-labeled data. However, high-quality labeled image datasets will always remain a vital component of this equation.
Conclusion
In conclusion, labeled image datasets are integral to the advancement of AI across numerous sectors. Investing in efficient data annotation tools like Keylabs.ai not only aids in the development of accurate models but also empowers businesses to make data-driven decisions that enhance their operations. As we look to the future, the importance of these datasets is only expected to grow, making it crucial for businesses to harness their potential today.