The Power of a Medical Dataset for Machine Learning in Today's Business Landscape
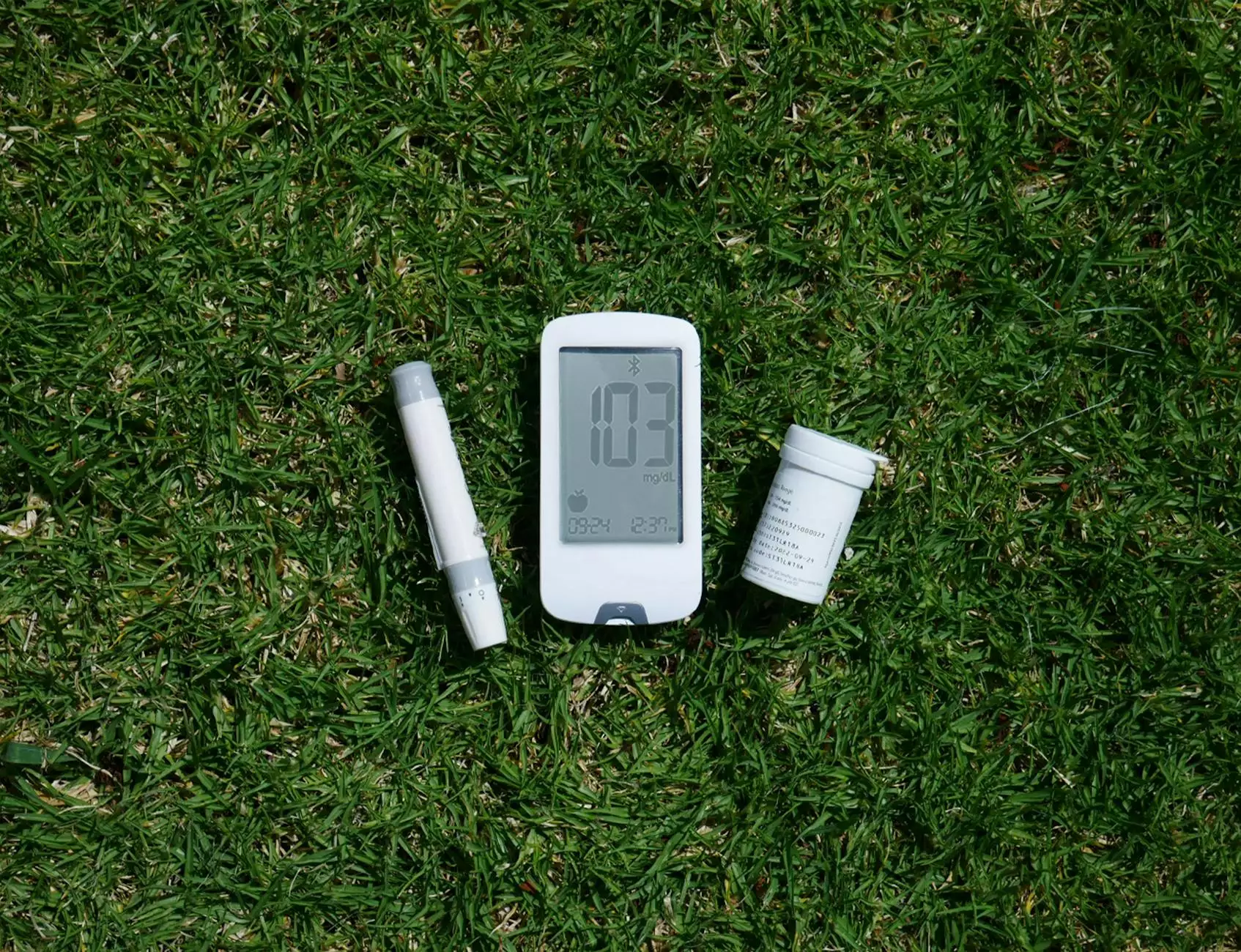
The healthcare industry is evolving at an unprecedented pace, significantly propelled by advancements in machine learning and artificial intelligence. One of the cornerstones of this transformation is the access to a medical dataset for machine learning. These datasets serve as foundational elements that can shape the future of healthcare businesses, from enhancing patient care to streamlining operational efficiencies.
Understanding Medical Datasets in Machine Learning
A medical dataset for machine learning is a curated collection of medical data that includes patient records, clinical trials, diagnostic results, and other relevant information. These datasets allow machine learning algorithms to identify patterns, make predictions, and assist in clinical decision-making.
Components of a Medical Dataset
- Demographic Data: Age, gender, ethnicity, socio-economic status.
- Clinical Features: Patient medical history, diagnosis, treatment interventions.
- Outcome Data: Treatment success rates, recovery times, side effects.
- Genetic Information: Genomic data relevant for personalized medicine.
- Real-time Monitoring: Data from wearables and mobile health applications.
Significance of Medical Datasets for Businesses
The integration of a medical dataset for machine learning into business operations can unrealize potential benefits and transformative changes. Here are the notable advantages:
1. Improved Patient Outcomes
Through predictive analytics derived from machine learning, healthcare providers can better anticipate health crises and enhance patient management. This proactive approach enables timely interventions that can drastically decrease hospital admissions and readmission rates.
2. Cost Efficiency
Businesses leveraging machine learning to analyze medical datasets can identify cost-saving opportunities. This could involve streamlining administrative processes or optimizing treatment protocols based on data-driven insights.
3. Personalized Medicine
Machine learning enables precision medicine by contrasting patient data with vast medical datasets. This leads to tailored treatment plans that align more closely with individual patient profiles, thereby improving treatment efficacy and satisfaction.
4. Accelerated Drug Development
In the pharmaceutical space, medical datasets for machine learning expedite the process of drug discovery. By analyzing clinical trial data and identifying potential drug interactions, companies can save time and resources in developing new therapeutic options.
Bridging the Gap: Integrating Machine Learning with Traditional Approaches
To fully harness the potential of medical datasets, businesses must integrate machine learning with traditional medical practices. This holistic approach ensures that data-driven insights complement the invaluable experience of healthcare professionals.
Best Practices for Implementation
- Data Quality Assurance: Ensure that the datasets used are accurate and up-to-date to yield reliable outcomes.
- Continuous Training: Adapt the machine learning models consistently as new data emerges or healthcare guidelines evolve.
- Collaboration Across Departments: Fostering a collaborative environment between data scientists and healthcare professionals can enhance the relevance of trained models.
- Regulatory Compliance: Adhere strictly to data protection laws and ethical standards, ensuring the responsible use of sensitive medical information.
The Future of Medical Datasets in Business
As we move towards an increasingly data-oriented healthcare environment, the role of a medical dataset for machine learning will only grow in significance. Emerging technologies such as blockchain for data integrity, IoT devices for real-time data acquisition, and advanced analytics tools will further enrich the capabilities brought forward by machine learning.
Factors Driving Future Innovations
- Technological Advancements: Innovations in artificial intelligence and big data analytics will continue to refine how we understand patient data.
- Growing Data Sources: Increased adoption of telemedicine and wearables will expand the pool of medical datasets available for analysis.
- Focus on Value-based Care: The shift towards value-based care models emphasizes the importance of patient outcomes and cost-effectiveness, driving demand for effective data utilization.
Case Studies: Success Stories in Utilizing Medical Datasets
Examining real-world applications of a medical dataset for machine learning highlights its effectiveness. Here are a few notable examples:
Case Study 1: Predictive Analytics at Hospitals
A large hospital network leveraged machine learning algorithms to analyze historical patient data. This initiative led to a 15% reduction in patient readmission rates by predicting at-risk patients and implementing follow-up care.
Case Study 2: Drug Development Acceleration
A pharmaceutical company used machine learning to analyze clinical trial data, shortening its drug development cycle by six months. By swiftly integrating findings from previous trials, the company successfully brought a new drug to market sooner.
Case Study 3: Personalized Treatment Plans
A healthcare startup utilized machine learning to create unique treatment plans for cancer patients. By analyzing genetic data alongside treatment outcomes, they achieved a significant increase in success rates for personalized therapies.
Conclusion: Embracing the Future
The business implications of medical datasets for machine learning are immense and transformative. As the healthcare landscape continues to evolve, businesses must adopt an adaptive mindset, embracing data as a strategic asset. Through informed decisions driven by insights from medical datasets, organizations can not only enhance patient outcomes but also ensure sustainable growth and innovation in a competitive marketplace.
Call to Action
For businesses looking to harness the power of medical datasets for machine learning, now is the time to invest in robust data infrastructure and analytics capabilities. By doing so, they can position themselves as leaders in the healthcare industry, ready to tackle tomorrow’s challenges with confidence.